Citizen Science Best Practice
Data Guidance
This is an interactive guide to explore the requirements for creating high quality data from a citizen science project. This work was funded by the Department for Environment, Food and Rural Affairs (Defra) as part of the marine arm of the Natural Capital and Ecosystem Assessment (NCEA) programme. Marine NCEA (mNCEA) is delivering evidence, tools and guidance to integrate natural capital approaches into policy and decision making for marine and coastal environments.
Introduction
The aim of the tool is to provide data guidance that can broaden the participation in environmental monitoring, maximise the efficacy of volunteers in citizen science projects so they can contribute good quality marine evidence which is FAIR. Ensuring that data are findable, accessible, interoperable and reusable maximises their impact and increases their longevity, as data users can find and easily use these data for research and data-driven policy, both nationally and internationally.
Citizen science, for the purpose of this guide, is defined as the involvement of volunteers in science; they can be involved at any stage of the scientific process. The guide describes volunteer projects in the widest sense, as the guidance may also be relevant to other non-volunteer projects.
The guide is divided into 6 main stages composed of a series of activities to consider within each stage in order to produce high quality, FAIR data. Each stage provides a list of data management recommendations which may be used as a checklist. To find data guidelines for a particular stage of a project, click on the relevant circle in the data lifecyle and then select the activity you wish to learn more about. Alternatively, click on the corresponding coloured dot on the matrix in the "Activities" tab for the activity and stage you want to view.
This guide can be viewed as the HTML transcript, or downloaded as a PDF:
This project was delivered by the Marine Biological Association of the United Kingdom, in partnership with Natural England, and funded by the Department for Environment, Food and Rural Affairs (Defra) through the Natural Capital and Ecosystem Assessment (NCEA) programme.
Activities
This matrix can be used to navigate directly to a specific activity within a specific stage of the data lifecycle. To do this, click on the coloured dot that corresponds to the activity and stage you wish to go to.
Stages | ||||||
---|---|---|---|---|---|---|
Data collection protocols | ||||||
Licensing and permissions for data collection | ||||||
Verification and quality control (QC) schemes | ||||||
Data collection tools | ||||||
Training | ||||||
Data standardisation | ||||||
Metadata | ||||||
Data processing tools | ||||||
Data verification | ||||||
Quality Assurance (QA) | ||||||
Data Archive Centres (DACs) | ||||||
Data flow pathway | ||||||
Licensing and permissions for data re-use | ||||||
Maintainance | ||||||
Controlled vocabularies | ||||||
Validation | ||||||
Data archiving | ||||||
Data publishing | ||||||
Social media | ||||||
Finding data | ||||||
Sustainability |
Plan
Overview
The first stage of the data lifecycle is the planning stage. A clear and thorough data management plan is recommended for any citizen science project that will collect and produce data. The plan needs to outline the expectations for collecting, using, and managing the data prior to proceeding to the collection events. Having a clear data management plan and consistently referring to it throughout a project promotes the integrity and consistency of the data produced and prevents future issues with data collection and publication. Data archive centres (DACs) can provide assistance with identifying standards, choosing a publication license, establishing a data flow pathway, and providing general data management support.
Activities
Data collection protocols
Following nationally and internationally recognised protocols and standards ensures that data collected by citizen scientists can be verified, replicated, and understood, maximising its usability in the future. Determining the protocols that will be used during data collection events during the planning stage, allows sufficient time and resources to be allocated for developing and testing of tools and resources as well as training staff and participants, reducing the risk of errors. Selecting sampling protocols at an early stage can also help to ensure that data forms can be designed to capture sufficient metadata at the sampling event to comply with standards and to be able to conduct a thorough quality assurance procedure at a later stage.
Specific protocols exist for many types of collection events. In the UK, UKDMOS contains many of the UK government agencies’ protocols and standards for marine monitoring programmes carried out in the UK and Europe. Specific projects and sampling techniques can be searched for in the directory, and the resulting metadata will provide references to standard protocols used, as well as when and where individual samples are collected. For example, JNCC's Guide to Fisheries Data Collection and Biological Sampling (Doyle, A., Edwards, D., Gregory, A., Brewin, P. & Brickle, P. (2017). T2T Montserrat; a guide to fisheries data collection and biological sampling. JNCC.) is referenced in UKDMOS. However, a wide variety of data collection protocols can be found in the directory. In instances where a protocol does not yet exists, existing standards may be used to guide the development of new protocols. If these are developed as part of governmental monitoring programmes, they can be added to UKDMOS here, or by contacting the relevant organisation hosting the standard protocols e.g. JNCC's Marine Monitoring Method Finder.
The sampling design may have an impact on the design of data collection tools and resources needed. For example, if a survey is conducted across a vast area e.g. different areas in the UK, using an automated data submission system would ease data submission at a later stage, rather than needing to compile paper records in one physical location. When a citizen science project intends to collect data at specific sites, it is beneficial to determine where the survey events will occur (spatial coverage) as well as when and where individual samples will be collected (spatial resolution). Research has shown that data from citizen science projects with greater spatial coverage, and an increased spatial resolution are used more widely in research as the data have a smaller size of minimum and major spatial units, facilitating their use in species distribution modelling and conservation planning. These data with large spatial coverage may be from a series of organised events at specific locations around the country, or projects where users choose where they wish to record data e.g. anywhere in the UK.
Recommendations
- Select a standard data collection protocol, if it exists, or if new protocols are developed, aim to follow exisiting standards, and outline this in the data management plan
- Determine the likely and required spatial coverage of the project
Data collection tools
Data collection tools can consist of mobile applications, websites, computer programs, and physical tools such as measuring devices, collection instruments, observation tools and identification guides. Things to consider with any chosen data collection tools are:
- How easy or difficult they are to use. The difficulty of using the tools will influence the training required to provide participants with an adequate proficiency to minimise errors during data collection. If the tools are very complex, it can also deter more casual participants from joining the citizen science project; however, offering in-depth training might alleviate these challenges whilst providing the opportunity for volunteers to upskill.
- Cost of obtaining and distributing the tools to volunteers. Depending on the funding available, compromises may need to be made, so how this may affect the data produced should be taken into consideration. For example, if a small number of identification guides, or GPS devices are to be shared among the participants, this is likely to affect the accuracy of the records so additional on-site verification of species identifications and coordinates may be required.
- If volunteers have to purchase their own tools. This may create a barrier to engagement for participants of lower socioeconomic status. Conversely, it may also increase repeated engagement from the same volunteers once they have the required tools.
- Risk of damage or loss at survey sites. A contingency plan should be devised in case of sampling equipment being lost or damaged to enable the data collection to continue, for example, having a few spares of tools that are most likely to be damaged, or limiting the use of these to staff and more experienced volunteers.
- Limitations of the tools. A common limitation when conducting marine and coastal surveys is reduced phone and internet signal. If this is an issue in the intended survey locations, a mobile application that operates off-line would be a priority. Similarly, tools need to be suitable to the environment of the survey, and people might not be willing to use their own personal electronic devices in the marine environment. For example, if collecting image data of species in a snorkel or dive survey, cameras must be waterproof to the appropriate depth and produce images of a high enough resolution to enable identification of taxa from the images.
- Accuracy and precision of the tools. This will include determining how precise the measurements need to be e.g. recording distances to the nearest cm or to the nearest m, and how accurate they are e.g. by calibrating measurement devices to ensure the measurements collected reflect the true value.
Recommendations
- Plan which tools are required, accounting for any training required for staff and/or volunteers to use them.
- Reflect on cost, accuracy, precision, and suitability to the environment.
Case Study
iNaturalist is a web-based platform which can be accessed via the website, or via a mobile application. It has been used for many past citizen science events such as The Rock Pool Project’s Changing Tides Bioblitz, the Time and Tide Bell project, and Crab Watch EU. When using the app to upload a sighting, a photo is taken through the app, and provided there is sufficient signal, an exact coordinate and time of sighting is recorded. It also is able to provide suggestions for the identification of the taxon based on the photograph taken, allowing users to compare their record to other images to identify the taxon. It is free, easy to use, and only requires users to have a smartphone, making it a popular tool choice for many citizen science projects.
Licensing and permissions for data collection
When organising a data collection event in which volunteers will participate, there may be restrictions associated with the sampling area. For example, if it is private land or a protected area, the relevant authorities or landowners should be contacted to reach an access agreement, and to receive prior approval for surveys and scientific monitoring. They may also provide specific rules which must be followed when accessing those areas which would need to be communicated to all participants prior to arriving on-site. Failure to obtain the required access permissions could result in an inability to use any collected data, or could prevent the data collection event from taking place. In addition to this, certain species require a license to be surveyed, further information on which species is available here.
Participants should grant permission for the data and media they collect to be used for research purposes, publications, reports etc and if the project wishes to take photographs to be shared, on social media for example, permission must be granted prior to the collection event. Participants may also take their own photographs or collect additional data which could be supplied to the project as supplementary data. The conditions of use should be agreed with the participants. In a permissions form, it may be useful to separate conditions for data/media access and reuse from permissions to use in social media and advertising as some participants may grant permission for data to be used in reports, btu not on social media, for example.
In addition to this, when conducting a thorough quality assurance, the data collector may need to be contacted to confirm details of the records, so some basic contact details for participants may need to be retained until the data has been through its QA. However, personal data should be limited to only collect what is relevant and necessary. If any personal data i.e. information that relates to an identified individual, is collected a plan must be in place to comply with GDPR guidance and regulations for data sharing under the Data Protection Act 2018. This will include ensuring personal data is processed securely, potentially by using pseudonymisation and encryption to enable data confidentiality, integrity, and availability.
Recommendations
- Plan and seek any permissions that may be required for the task, including access permissions from landowners, consent from participants to use the collected data and media such as photographs for analyses, research and publication and whether these can be used for social media and advertising purposes.
- Plan the creation of permission forms for the use and storage of personal data to comply with GDPR regulations, keeping this to only data that is necessary.
Case Studies
Lundy Marine Protected Area
Lundy Marine Protected Area provides guidance on the website on permitted activities, and those that require anyone wishing to conduct surveys and scientific monitoring to consult with the warden. The warden could also be contacted to determine whether there are specific requirements to share data with the Lundy Marine Protected Area upon completion of the project, and whether they require acknowledgment in publications created from these data.
Marine Biological Association Bioblitzes
The Marine Biological Association has run Bioblitzes since 2009. A Bioblitz is a short-term data collection event in which participants aim to record as much as they can within a set area to provide a snapshot of biodiversity in that area. Participants were asked to complete and sign a permissions form granting the use of any photography taken at the event in future publicity and social media posts linked to the event. Additional permissions can be requested to this sort of form such as permission to use images taken by volunteers for future species verification. An example image permissions form from the MBA.
Use of Images
By contributing photographs (and/or video) to the Data Desk at the MBA Bioblitz, you have agreed that your images/ videos can be used to support records submitted during the Bioblitz event during [insert date]. Please delete as appropriate which use(s) of your photos which you give permission. Contributors retain the copyright of their images.
Photographs and/or videos may be used (please delete as appropriate):
- For promotional use for future MBA public events: YES / NO
- In documents relating to the event (e.g. report and website): YES / NO
- On the MBA Social media pages: YES / NO
- In posters and/or presentations associated with the MBA Bioblitz: YES / NO
If images are required for commercial purposes or for wide dissemination, the image provider WILL be contacted for permission directly and in the form of an acknowledgement obtained from the owner.
Image provider details
In order to ensure that we can contact image providers, please provide the following contact details.
Full name: ………………………………………………………………………………………………………………………………………………………
Contact address: ………………………………………………………………………………………………………………………………………
Email address: ……………………………………………………………………………………………………………………………………………
Image provider permission
I agree to the use of my images as specified in the above terms and conditions
Full name: ………………………………………………………… Signature:……………………………………………………
Date: ……………………………
Verification and quality control (QC) schemes
Data verification methods to be conducted at the collection event and any relevant quality control (QC) schemes should be determined within the data management plan. Verification can involve the checking of records at the site by subject experts to ensure records are accurate and to reduce the risk of species misidentification, or the incorrect recording of coordinates. A trial collection event conducted by professionals carried out prior to the citizen science event can help to mitigate errors and improve accuracy by identifying potential risks, developing further training where necessary, and providing a baseline data collection standard to which the citizen science data can be compared.
Data verification following a data collection event ensures that data and taxa identifications are accurately recorded. Verification processes can be built-in to the platform where data will be recorded, for example iNaturalist and iRecord, or it may need to be planned separately if the citizen science project intends to publish records via a different route, e.g. as a whole survey to a data archive centre. This could involve collecting samples at the site of data collection and having an expert in that taxonomic group identify the taxa at a later date. Another example of data verification is the process the Zooniverse uses in projects where images are analysed by multiple volunteers. This differs from data validation, which may involve automated processes to validate that data are in the correct format and meet the required data standards.
The NMBAQC scheme describes the importance of collecting a voucher/reference collection when recording biodiversity data to be used as a reference for future identification of taxa or habitats. This voucher specimen can be a preserved specimen, or clear images of the specimen or habitat.
Another quality control method is to repeatedly sample the same area and obtain an average of results, which may be more suitable for projects where the sampling area is relatively small and there are sufficient participants to repeat sampling. Quality control differs from quality assurance (QA), as QC involved checking the data for errors e.g. bias and systematic errors, and recorded correctly prior to submission to a DAC, whereas QA assesses the data are correct, accurate, in the correct format and that it meets data standards, and may include a validation step prior to publication, and this step may be carried out by the DAC which will archive and publish the data.
By outlining the verification and QC methods which are expected to be carried out at the data collection in the data management plan, the methods can be standardised across survey sites and any additional training, equipment or staff required can be planned well in advance.
Recommendations
- Outline any on-site and post-collection data verification methods and data quality control (QC) schemes in the data management plan
Training
Participants will need the appropriate skills to conduct the tasks required during the citizen science project. Outlining the training requirements in the data management plan enables sufficient time and resources to be allocated for training participants, both volunteers and staff, before starting to collect data. Training sessions may focus on the preparation for the event, what to do during the event or how process the data following the event. Training must be tailored to the complexity of the data collection protocols, equipment used and the experience level of the participants. Training may occur before, at the start of a data collection event, or after if focused on data management. The following elements should be considered when planning training resources:
- Complexity of collection methods. Complex sampling methods will require more extensive training thus benefiting from closer supervision by either professional scientists or volunteers with previous experience with similar techniques. Complex methods are also more likely to incur errors, so reducing the complexity as much as possible reduces the risk of bias and systematic errors. This can be achieved by breaking down complex tasks into easier tasks, e.g. when recording species in biodiversity surveys, splitting the volunteers into groups and allocating a subset of species for them to record allows them to become more familiar and skilled at identifying those taxa.
- Equipment used by volunteers. Similar to the complexity of collection methods, equipment that is more difficult to use will require further training and supervision by those who are more skilled in the use of the collection tools.
- Experience level of citizen scientists. Volunteers that have completed more advanced training, are likely to produce more high-quality data. Less experienced participants will require more comprehensive training. A buddy or group surveyor system can be beneficial for surveying events where there is likely to be a range of abilities, pairing experienced participants with less experienced volunteers.
- Standardisation of training resources and programmes. If the project is to be carried out in different locations, the training provided to participants should be consistent across locations to ensure the data collected is of the same quality. This can be achieved by having an overarching training curriculum followed by all those providing training to volunteers, using the same training resources wherever possible. Online training sessions are an effective way of delivering training to people in different locations, or funding an in-person workshop in a centralised location. Prior testing participant abilities can improve reliability but can be a barrier to participation if requiring significant learning time.
Training materials can consist of pre-collection training such as courses (online or in-person), assignments to get participants familiar with the collection methods and the data handling required, video tutorials, identification guides and survey technique guides.
Recommendations
- Outline training in the data management plan, considering complexity of data collection protocols, equipment used, experience level of participants and the requirement for standardisation of training materials.
Case Study
SeaSearch is a citizen science project that aims to record marine habitats and species around the UK coasts. The training programme is split into four levels of progressing difficulty, encouraging volunteers to progress through the training as they become more experienced surveyors and giving an incentive to continually participate in surveys to improve their skills. Courses consist of either online or in-person sessions, and completing practice survey forms. Identification guides and training materials are included in the cost of each course, so volunteers can review the resources at any time. Trainees must then put the skills to practice by carrying out qualifying surveys supervised by a tutor. Once qualified, the volunteers are able to join as many or as few surveying events as they like, and have the opportunity to continue more specialised training if they wish to do so. As SeaSearch relies on trained divers and snorkellers, survey training and support is a crucial part of the project.
Data standardisation
Data standards are a set of rules for formatting data to make them consistent across datasets. Complying with data standards enables the data to be interoperable and reusable, so they can be shared with national and international data aggregators, used for a wide variety of work. Being interoperable and reusable means the data has a greater impact as they can be used in environmental monitoring programmes, they can inform essential biological variables (EBVs) and essential ocean variables (EOVs), and can contribute to initiatives like the UN Ocean Decade, as well as the Marine Strategy Framework Directive.
Data standards should be determined prior to data collection as this will help to identify which data fields are mandatory to complete in order to comply with the standards e.g. survey date, survey location, sampling devices. Doing this early on means that you will be able to ensure this important information is captured as standard from the beginning of the project.
The standards selected will depend on the type of data collected and the theme it falls under. In the UK, MEDIN is the network of marine-focused data archive centres that contribute to the creation of marine data standards and implement the adherence to these. MEDIN standards are categorised by marine data theme e.g. biodiversity, oceanography, meterology, with templates and data guidelines that data providers can complete to ensure their data are compliant by completing all mandatory fields. Data that are MEDIN.compliant are also GEMINI (national) and INSPIRE (global) compliant, which means they comply with national and international standards. These data can also then be transformed into other data standards such as DarwinCore when published to enable interoperability and the harvesting of data by aggregators such as OBIS. Other common data standards are Ecological Metadata Language and BioCASe / ABCD.
Recommendations
- Identify appropriate data standards for the data being collected and determine which mandatory information needs to be recorded during the data collection event. If data are to be submitted to a MEDIN DAC, choose the appropriate MEDIN data guideline.
Metadata
Metadata is data that provides information about other data. It provides information to data users which help them to understand the data and how they can be used without needing to contact the data provider. When a dataset is published, a metadata record should also be published alongside it. A metadata record provides detailed information such as who owns the data, any access and reuse restrictions, any unique identifiers for the dataset, the date it was collected and published, where it was collected, where to access it and who to contact if there are any questions about the dataset. Metadata records should also comply with standards. To produce a high-quality metadata record the standards should be researched prior to collection events to ensure all mandatory information is recorded. MEDIN metadata records comply with the MEDIN Discovery Metadata Standard, and are published on the MEDIN Discovery Metadata Portal, where users can search for datasets and their metadata.
Recommendations
- Research metadata standards in the planning stage to ensure all mandatory information is collected in the data collection and processing stages.
Data processing tools
Once the data have been collected, they may need to be processed to put them into a format which can facilitate data standardisation, quality assurance and publication. This can involve digitising paper records, scanning documents, or identifying taxa from photographs and videos. The processing steps should be outlined in the data management plan along with any tools required. This will enable sufficient training and resources to be allocated for effective processing of the data. For example, if data will be processed using an automated script, personnel must have the appropriate skills and software to do this. Examples of data processing tools may include Microsoft Excel, Python, R package., QGIS, or coordinate conversion scripts.
Recommendations
- Define data processing tools in the data management plan to allocate appropriate training and resources.
Quality Assurance (QA)
Quality assurance (QA) of data and metadata is carried out after any data processing, and aims to identify and correct any mistakes that may exist in the data produced by a project. The main areas that should be assessed during the QA of a dataset are spatial accuracy, taxonomic accuracy, methodological consistency, and temporal accuracy. Adherence to the selected data standards is also checked during the quality assurance of data and metadata, prior to validation of the dataset against specific standards to minimise corrections required at a later stage. When submitting data to a data archive centre, as much information as possible should be provided to the DAC so they can carry out a thorough QA of the data. This information can include any raw data, including original paper records if relevant, any images collected, GIS files, videos, contact details of the data provider, any relevant documentation such as project reports, vessel reports or logs. By establishing the QA procedures at an early stage in a citizen science project, a checklist can be made of information that must be retained for effective QA of the data.
When quality assuring the data and metadata, a variety of tools could be used, such as Microsoft Excel formulae, R package., taxon match tools, e.g. WoRMS and MSBIAS, coordinate converters, geographic mapping software like QGIS or Google Earth. If submitting data to a data archive centre like DASSH, you may not need to conduct the quality assurance yourself as they may have their own quality assurance procedures. The relevant DAC should be contacted to discuss data requirements for conducting their QA procedures. A list of MEDIN data archive centres and their contact details can be found on the MEDIN website.
Recommendations
Data Archive Centres (DACs)
Data archive centres (DACs) archive and publish data, making them available for a wide range of users and data aggregators to harvest and use. They can also provide data-focused leadership, best practices, services, tools, and training to support the archiving and publication of data in a standardised format. This ensures data is findable, accessible, interoperable and reusable, or FAIR.
MEDIN, the Marine Environmental Data and Information Network, consists of 7 data archive centres which specialise in archiving different types of data from fisheries, water column oceanography, meteorology, bathymetry, historic environment, geology and geophysics, flora, fauna and habitats.
The relevant DAC should be contacted during the planning stage to be well informed of the data submission process, standards used, and any further requirements. If you are unsure about which DAC the data from your citizen science data should be submitted to, or have any questions, you can contact the MEDIN team at enquiries@medin.org.uk.
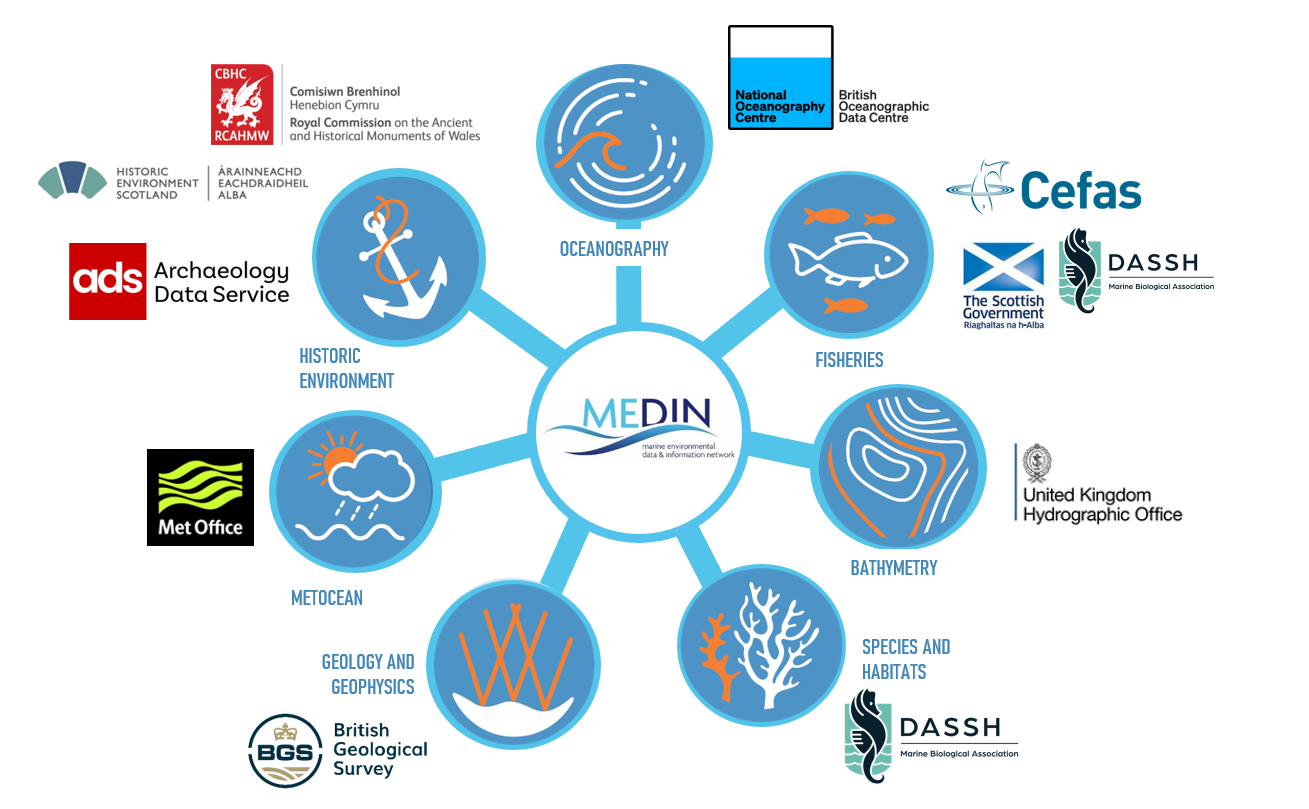
If the citizen science project has received funding, it is possible that the archiving and publication of any data produced to a DAC is a requirement. Even if this is not the case, it is still strongly recommended to do this to ensure data are FAIR and their longevity is maximised well beyond the lifespan of the citizen science project.
Recommendations
- Specify the data archive centre you will archive and publish data through in the data management plan.
- Contact the data archive centre at an early stage to receive specific guidance relevant to your data.
Case Study
The Marine Biological Association and the Natural History Museum established the Mitten Crab Watch project to encourage citizen scientists to record sightings of the invasive non-native species, the Chinese mitten crab (Erocheir sinensis). In the past, the MBA hosted a website for submitting sightings which shared records to the Biological Records Centre, which in turn shares data with NBN Atlas. The MBA no longer organises citizen science activities directly relating to this project, but provides support and guidance for those wishing to find out more about Chinese mitten crabs and submit their sightings.
Data flow pathway
The knowledge that their data will be contributing to science, public information and conservation can motivate citizen scientists to take part in a project. Determining the pathway the data will take once collected, and communicating this to potential volunteers when advertising the event could also increase the number of participants and the quantity of data that can be collected.
The type of data recorded by citizen science projects, as well as the intended degree of sharing of the data will influence the pathway those data will take. For example, single records submitted by users of iNaturalist will follow the route in Figure 2. These data are more likely to be collected by casual citizen scientists recording ad-hoc sightings, but individual record submission platforms like iNaturalist and iRecord can also be used to aid data submission from organised data collection events. Larger datasets are more likely to be archived and published by data archive centres like DASSH (marine biodiversity and habitats data); the pathway these data take from DASSH are also shown in Figure 2.
Figure 2: DASSH data flow
Recommendations
- Determine the route the data will take once collected, considering whether they will be submitted as individual records or whole datasets.
- Plan to communicate the data pathway clearly with citizen scientists to increase future engagement.
Case Study
The Rock Pool Project has been clear with volunteers regarding the pathway their data travels through, communicating this via training presentations prior to their citizen science events. Figure 3 shows the flow diagram they have shared with participants.
.png)
Licensing and permissions for data re-use
All high-quality data produced by a citizen science project should aim to be FAIR: findable, accessible, interoperable and reusable. This can be achieved by complying with data and metadata standards, making these openly available to users in an interoperable format, and as openly accessible as possible. By researching data licenses and reuse conditions at this stage, the data pathway and collaboration with data archive centres can be started at an early stage, and to liaise with funders and data owners on how the data will be shared upon completion of the project or collection event.
If volunteers are collecting and submitting data directly (e.g. through a mobile app) then you may want to consider providing guidance/training on data licensing and how to select the appropriate license to ensure maximum usability.
Recommendations
- Outline any conditions to data sharing, access and reuse the data produced by the citizen science project will have in the data management plan.
Maintainance
After a dataset is published or archived, data maintainers will be responsible for making amendments to the dataset itself or to the associated metadata. This may occur if the ownership of data changes, if the title changes to match a publication, if amendments are made to records, or if the further surveys are carried out in the future which are part of the same project and so can be linked together via a series. If the data are published via a data archive centre, an agreement can be made for the DAC to be responsible for the updates, or the data owners or other named organisation can carry out any updates. To ensure data remain accurate and reusable, it is important that the maintainers are specified when data are archived and published.
Recommendations
- Plan how data resulting from the project will be maintained and by who, should the project end, corrections be required, or additional data be added at a later stage.
Case Study
The MBA.s Bioblitz.s have been carried out regularly since they began in 2009. The Bioblitz events form part of an overarching series (see metadata record here), so as new bioblitzes are carried out, their metadata can be linked together in this parent series metadata record. As DASSH is the DAC which archives and publishes the MBA.s bioblitz data, in this case DASSH is also responsible for making any changes to the series metadata record, the DOI., and the data themselves if this is required.
Summary & Checklist
A citizen science project that produces high quality, FAIR data, should cover the following points in the data management plan:
- Select a standard data collection protocol, if it exists, or if new protocols are developed, aim to follow exisiting standards, and outline this in the data management plan
- Determine the likely and required spatial coverage of the project
- Plan which tools are required, accounting for any training required for staff and/or volunteers to use them
- Reflect on cost, accuracy, precision, and suitability to the environment
- Plan and seek any permissions that may be required for the task, including access permissions from landowners, consent from participants to use the collected data and media such as photographs for analyses, research and publication and whether these can be used for social media and advertising purposes
- Plan the creation of permission forms for the use and storage of personal data to comply with GDPR regulations, keeping this to only data that is necessary
- Outline any on-site and post-collection data verification methods and data quality control (QC schemes in the data management plan
- Outline training protocols, considering complexity of data collection protocols, equipment used, experience level of participants and the requirement for standardisation of training materials
- Identify appropriate data standards for the data being collected and determine which mandatory information needs to be recorded during the data collection event. If data are to be submitted to a MEDIN DAC, choose the appropriate MEDIN data guideline
- Research metadata standards in the planning stage to ensure all mandatory information is collected in the data collection and processing stages
- Define data processing tools in order to allocate appropriate training and resources
- Outline the quality assurance procedures to determine which data need to be retained for effective data QA
- Contact DAC to establish whether they have their own quality assurance procedure and if so, what information they require to conduct a QA of the submitted data
- Specify the data archive centre you will archive and publish data with
- Contact the data archive centre at an early stage to receive specific guidance relevant to your data
- Determine the route the data will take once collected, considering whether they will be submitted as individual records or whole datasets
- Plan to communicate the data pathway clearly with citizen scientists to increase future engagement
- Outline any conditions to data sharing, access and reuse the data produced by the citizen science project will have
- Plan how data resulting from the project will be maintained and by who, should the project end, corrections be required, or additional data be added at a later stage
Collect
Overview
During the data collection stage of a citizen science project, the following elements should be considered: collection methods and tools required, participant training and experience levels, planning of the collection event, data collection verification and quality control.
Activities
Data collection tools
To produce high-quality data, the equipment will need to be standardised and calibrated across volunteers and staff. Iterative development and design of the tools required, i.e . refining the tools required for volunteers through a few rounds of ‘test’ collection events to identify the limitations and potential systematic errors using certain tools may incur. Further information on iterative design of tasks and tools can be found here.
The data could be collected by using handheld devices such as a smartphone or laptop, they could be recorded manually on paper, or use a combination of both, where citizen scientists may initially record data manually but they submit these data online. Whether the collection method is manual, hybrid or virtual will affect which tools are required. Large-scale projects which require volunteers across a large area to submit records in a given time frame may benefit from using a hybrid or virtual collection with online tools, whereas smaller scale, supervised projects may benefit from recording taxa manually, then collecting and verifying the manual records before digitising them for collation and submission to a DAC for publication.
The tools required will also depend on the type of data the project intends to collect. For example, whether this will consist of taxonomic classifications, percentage cover estimates, presence-absence determinations, counts, organism trait measurements or environmental measurements. A rock pooling survey where volunteers record the presence of all organisms encountered within the survey may only require the use of a recording app, or a sheet of paper along with an identification guide. However, a project that wishes to conduct transect surveys along a coastline to measure environmental and biodiversity variables like shore cover and taxon abundance might require the use of distance measuring devices, quadrats, as well as the recording tools like cameras, paper or handheld devices. In both cases, the tools should be standardised, calibrated, and assessed for suitability to the environment and skill level of volunteers.
Examples of data collection tools that may be used in a citizen science project include
- Manual recording materials e.g. notebooks, pre-designed worksheets to be handed to volunteers.
- Virtual recording materials e.g. mobile phones, apps, computer programs such as Microsoft Excel, websites such as iRecord, online forms, cameras.
- Measuring tools e.g. measuring tapes, GPS devices, quadrats, transect lines.
- Specimen collection tools e.g. nets and traps.
- Guides e.g. identification guidebooks, virtual guides, identification software e.g. like the species identification software in iNaturalist.
Recommendations
- Select tools which are standardised and calibrated across users, and suitable to volunteer skills and the environment in which they’ll be used
- Test tools at the survey location prior to the collection event to determine potential issues and errors
- Tailor the tools used to the type of data that will be collected and the scope of the project
Case Study
Shark Trust
The Shark Trust run a citizen science project called the Great Eggcase Hunt, which aims to encourage citizen scientists to hunt for eggcases on beaches around the world and recording what they find. They have developed an app for users to upload sightings, as well as allowing users to easily upload sightings to their website. The app combines five citizen science projects led by The Shark Trust: the Greate Eggcase Hunt, Shark Sightings Database, the Basking Shark Project, Entanglement Survey, and the Angling Project. As users submit their findings, they build up a list of their research contributions which are saved to their profile and shared with the wider community. This tool is very easy to use which encourages participation from a wide range of volunteers.
Data collection protocols
The data collection methods should be appropriate for the expected experience level of the citizen scientists, with relevant training and tools provided to ensure the data is collected accurately to produce high-quality data. The methods used will depend on the survey type e.g. transect, quadrat, seine-netting, pond-dipping, rock pooling, mammal trapping, walkovers, push-netting, grab/core sampling, dive or snorkel surveys, timed species-searches, plankton netting. These collection methods might be carried out by volunteers, professionals, or a combination of both.
To ensure data collected are of a high quality, trial collections are recommended, where professional or experienced surveyors conduct the collection protocols using the selected tools to determine where systematic errors or difficulties are likely to occur. This allows for the protocols to be developed further to reduce the risk of these errors, or to modify the processing of the data to adjust for systematic bias e.g. calculating mean abundances over a few groups of volunteers. In addition to this, previous research has highlighted that with adequate training and low-taxonomic-resolution protocols, citizen scientists can generate data similar to those of professional scientists.
Standardised methods for data collection are available to ensure data collected is interoperable and reusable. An extensive list of standardised methods is available here, with a list of protocols and standards for UK and European marine monitoring available on UKDMOS.
Recommendations
- Select standardised data collection protocols which are tested by professionals prior to being carried out by volunteers.
- Allow adequate time for training and testing the protocols alongside data collection.
Case Study
Seasearch Observations/Surveys
SeaSearch volunteers record their sightings by submitting a form that is completed following a dive or snorkelling survey. The survey may be a planned survey where the volunteer records what they see along a dive, both biodiversity records and geographical information of the site, or it can be a recreational dive in which a diver spots specific taxa which they wish to submit sightings of. Depending on the surveyors experience level they may complete an observation form or a survey form, which is a little more detailed. Both forms record important metadata that is essential to produce high-quality data. This includes the coordinates of the dive site, recording start and end points of the dive/snorkel, how the position was determined (GPS/chart/ordinance survey map) date, time, max depth, sea temperature, visibility, general habitat descriptions, and species seen. Detailed guidance notes are also provided to help volunteers understand why they need to record certain information, and how to do so. The techniques taught and materials provided by SeaSearch for all volunteers are standardised across locations, and volunteers are tested on their data collection skills prior to being able to submit their own data.
Verification and quality control (QC) schemes
Verifying data that is recorded at the collection event and conducting quality control on this and the data themselves is recommended to produce accurate and reliable data of a high quality. The amount of on-site verification and quality control (QC of data will depend on citizen scientist skill levels, design of collection procedures, equipment, expert verification, replication across volunteers, accounting for random error and systematic bias. Verification may involve having experts verify taxonomic identifications and locations prior to submitting records or checking small samples of data collected by volunteer groups to ensure key taxonomic groups have been identified correctly. Checking samples of data from individuals or groups of volunteers is also likely to reduce the effects of inter-observer variability, especially where data recorded consist of estimates rather than absolute numbers e.g. percentage algal cover in an intertidal quadrat survey. This differs from validation, where automated procedures may be used to check the data format and its compliance with the desired data standards/
Recommendations
- Have a data verification or quality control procedure in place to check records for accuracy at the data collection event.
Case Studies
iRecord is a website for sharing biological observation records. When submitting records, automatic verification is carried out to help spot any errors, and then experts carry out a verification procedure to ensure identification of records are accurate. Most iRecord verifiers are expert volunteers working on behalf of national recording schemes, who specialise in certain species groups or specific geographic areas. Further information on iRecord.s verification scheme can be found here.
MBA Bioblitz
Bioblitz events run by the Marine Biological Association had a number of experts at the survey site to verify species identifications on-site and to assist with collection protocols ensuring data was accurate, e.g. 2016 MBA Plymouth Hoe Bioblitz. Detailed guidance on how to run a Bioblitz can be found on The Natural History Consortium's website, and in a guide produced by The Natural History Museum.
The Rockpool Project's Bioblitz in October 2023 also had experts on-site to verify records, and made use of iNaturalist.s record grading system to only accept records which were classed as "Research Grade", meaning that they contain a valid date, location, photo or sound, isn't a captive or cultivated organism, has been identified and that identification has been reviewed and the iNaturalist community agree with this identification.
Shoresearch
Shoresearch is a national citizen scientist project run by The Wildlife Trusts that aims to train volunteers to identify and record the wildlife found on the UK shores. Shoresearch survey techniques are standardised across the UK, with data initially verified on-site by experts from The Wildlife Trust. The data are collected on standardised paper recording forms or the Shoresearch app, and then uploaded onto the national Shoresearch Portal website. The website is custom designed for simple use, with data hosted by the Biological Records Centre. From here, the aim is for the data to be verified via iRecord's verification system and automatically exported to the National Biodiversity Network, though this last step is still in development. You can find out more about Shoresearch's data processing here, as well as their terms and conditions and privacy policy.
Licensing and permissions for data collection
At the data collection event, permission to use images of and data collected by volunteers should be obtained if not already received prior to the event. If data is to be collated into one survey dataset which will be submitted to a DAC, and no personal details of the volunteers are supplied in the dataset, it will be the responsibility of the owner of the data, i.e. for the whole project, to select a suitable license. However, if volunteers are submitting their own data to a website or database from a personal account, they must select the license under which they wish to share their data to enable its reuse in the future.
Permissions for accessing a specific survey site should be determined prior to data collection, in the planning stage. At the collection event, any restrictions or rules of access should be communicated clearly to all volunteers.
Recommendations
- Ensure volunteers have signed relevant data use permission forms or selected licenses for their sightings if submitting data individually.
- Communicate restrictions resulting from obtaining permission to access specific survey sites to all volunteers.
Case Study
iNaturalist accounts automatically set the license for any sightings that are submitted as CC-BY-NC, which means that users are free to share and adapt the data so long as attribution is given and it is not used for commercial purposes. However, users may amend the license given to their sightings in their account to change the terms under which their records are used and shared. By selecting an open license type such as CC-BY and CC0, data are accessible and reusable, supporting FAIR principles.
Training
Participants should have the appropriate skillset for the work they will be carrying out during a citizen science project1. This will involve providing any relevant training resources prior to, during, and after the data collection event as well as any relevant tools, like recording apps such as iNaturalist.
Training can also be tailored to the experience level, providing more advanced training to those who are more experienced and encouraging citizen scientists to progress through the training programme and develop their skills. The experience level of surveyors can be categorised, from most to least experienced, as professional, academic, naturalist, volunteer with expert ID, or volunteer. Buddy systems can be used during the data collection to allow more experienced surveyors to check the work of newer volunteers and to develop their training further.
In general, the method of data collection can either be carried out by volunteers and classified by professionals, carried out by professionals and classified by volunteers, or both collected and classified by volunteers. Each of these methods will have different training requirements to maximise data quality. For example, if data are collected by volunteers and classified by professionals, training should focus on the use of measuring devices and recording equipment such as cameras, as professionals would conduct the analysis and classification of records. If the inverse occurs, training for volunteers would also need to focus on taxonomic identification.
1 Kosmala, M., Wiggins., Swanson, A. and Simmons, B. (2016). Assessing data quality in citizen science. Frontiers in Ecology and the Environment, 14(10), 551-560. doi:https://doi.org/10.1002/fee.1436
Recommendations
- During data collection, ensure all volunteers have received appropriate training to carry out the work required, considering experience, tasks and tools required, and difficulty.
Case Studies
ORCA
The Marine Mammal Surveyor course run by ORCA uses a combination of online and in-person training as well as supervision from more experienced surveyors while out on surveys. Prior to taking part in any surveys, participants must attend a day-long online course consisting of identification methods for cetaceans, survey techniques and practice exercises to fill out survey forms. During surveying events, volunteers consist of a mix of experience levels to allow experienced surveyors to assist and train less experienced individuals. Physical identification guides are provided, as well as all tools required e.g. binoculars, access to the vessel's GPS.
Seasearch
The SeaSearch training programme is available at three levels depending on experience: Observer, Surveyor and Specialist. A novice would start training as an Observer, and as their skills progress they can then complete training for Surveyor and Specialist courses. Trainees must complete supervised surveys which are assessed by SeaSearch trainers to demonstrate their skills are adequate for the surveys they conduct. This system encourages volunteers to keep working with SeaSearch as they can improve their skills over time, becoming more reliable surveyors as their experience increases, producing robust data.
iNaturalist
As iNaturalist allows citizen scientists to upload record observations via the app or their website at any time, rather than during a specific sampling event, video guidance is provided on the website to explain how upload sightings to iNaturalist. When submitting sightings, there is also clear guidance within each field so help recorders know which fields are mandatory and how to complete them.
Summary & Checklist
When running a citizen science data collection event, such as a biodiversity survey, the following should be considered:
- Select tools which are standardised and calibrated across users, and suitable to volunteer skills and the environment in which they’ll be used
- Test tools at the survey location prior to the collection event to determine potential issues and errors
- Tailor the tools used to the type of data that will be collected and the scope of the project
- Select standardised data collection protocols which are tested by professionals prior to being carried out by volunteers
- Allow adequate time for training and testing the protocols alongside the data collection
- Have a data verification or quality control procedure in place to check records for accuracy at the data collection event
- Ensure volunteers have signed relevant data use permission forms or selected licenses for their sightings if submitting data individually
- Communicate restrictions resulting from obtaining permission to access specific survey sites to all volunteers
- Ensure all volunteers have received appropriate training to carry out the work required, considering experience, tasks and tools required, and their difficulty
Process
Overview
Following the data collection, data should be verified, standardised, quality assured, and validated to ensure the data are interoperable and reusable so they can be used for a wide range of further analyses. The appropriate standards and controlled vocabularies need to be selected for the data types recorded and for the intended data sharing platform, as outlined in the data management plan.
Activities
Data Archive Centres (DACs)
Data archive centres can aid with the standardisation and quality assurance of citizen science data. They may require datasets to be submitted in a specific format such as the MEDIN guidelines, in order to conduct quality assurance free of charge, or they may be able to provide data digitisation and standardisation services if required. The DAC responsible for archiving the relevant data type should be contacted at an early stage to discuss what services will be required from them and the data they will need you to provide in order to perform these services.
Case Study
DASSH (The UK Archive for Marine Species and Habitats Data) will create a data DOI, archive, QA and publish marine biodiversity data free of charge under the condition that the data are provided in MEDIN Guideline format. Any raw data, logs, reports, original GIS data etc., also need to be supplied for the data to be checked against this original data. Once the data are provided in this format, DASSH can proceed with quality assurance of the data set against the raw data and the MEDIN guidelines. DASSH also require data providers to create a MEDIN metadata record which will enable discovery of the dataset on the MEDIN Portal once published. This metadata record should not be published by the data provider; DASSH will also quality assure the metadata record and publish it with the data download link once the dataset has been quality assured.
Data verification
The data verification in the processing stage is carried out following data collection, and aims to check any records for accuracy and reliability. This is particularly recommended if on-site verification is not possible, for example for citizen science projects where volunteers collect data on an ad-hoc basis rather than as part of a group in an organised event. Where mobile apps and web forms are used to submit data they may redirect records to a verification system where expert verifiers can confirm taxonomic identifications, and then have automated integrated data validation systems to ensure records contain all the mandatory information prior to submission. Data archive centres can also verify records by contacting experts in specific taxonomic groups to verify species identifications and their geographical ranges. Verifying records prior to processing the data further reduces the risk of taxonomic and geographical errors in the data, producing accurate and reliable data. This differs from validation where automated processes may be used to ensure data are in the correct format and comply with the specified data standards.
Recommendations
- Ensure records are verified prior to further processing to increase data accuracy and reliability.
Case Study
Records can be submitted to iRecord directly through the website, or via iNaturalist. iNaturalist records which have had their taxonomic identification confirmed by two or more naturalists, and have all mandatory metadata, flow through to iRecord for expert level verification. iRecord has a series of automatic checks based on rules about how difficult the species are to identify, whether they have been recorded within their known distribution, and within its regular period of activity. The automatic checks help expert verifiers to identify which records may need further examination prior to submission. The records are initially marked as “Unconfirmed: Not reviewed” before experts confirm whether they are accepted, or not accepted, as shown in figure 4. The experts are usually volunteers with a specialty in specific taxonomic groups who work on behalf of the national recording schemes. This automated identification of records and the manual verification step increase data quality and ensures records are suitable for use in research, planning and conservation.
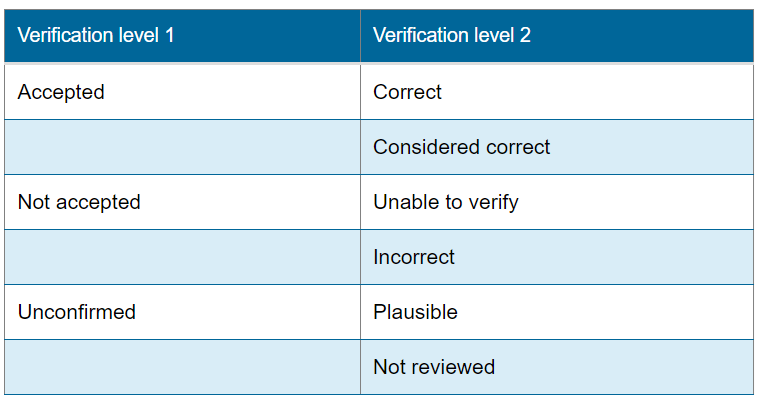
Data standardisation
Data standards are best practice guides for archiving marine data. They provide a framework of information that should be collected with your data to ensure that they can be reused by others in the future. Standards allow information to be captured at the time of data collection or immediately afterwards, so they can provide a checklist of essential information to collect. They instil good practice amongst users as they provide a standard format to work to and allow easy ingestion of data into data archiving centres. Some DAC. like DASSH will be able to publish data for free if it is submitted in one of the data standards such as MEDIN or Darwin Core. Data submissions in non-standard formats is possible, but there may be cost involved for transformation.
Standardising data involves converting the raw data into a format that is consistent with specific data standards, so that it is interoperable and reusable. Standardisation of data and metadata allows users to understand data effectively without needing to contact the data provider, reducing the risk of misinterpretation, saving time and resources used to reformat data into a usable form. This vital step also enables onward publication or sharing with national, regional and global data aggregators, increasing its impact by allowing more users to find and access it, supporting FAIR data principles.
Common data standards for biodiversity data include:
- MEDIN guidelines. MEDIN produce standard guidelines for a range of marine environmental data themes. Data that are compliant with MEDIN standards, are also compliant with UK GEMINI, EU INSPIRE Directive and ISO 19115 and 19139 data standards.
- Darwin Core. Data submitted to NBN Atlas, OBIS and EMODnet must be provided in Darwin Core format, which is an international standard for biodiversity data.
- ABCD is the Access to Biological Collections Data exchange schema, a biodiversity data standard for the access to and exchange of data about specimens and observation.
- Other marine data standards are listed on the MEDIN website.
Metadata standards include:
- MEDIN Discovery Metadata Standard. This metadata standard is also compliant with GEMINI and INSPIRE standards. Compliant metadata records are published on the MEDIN Portal.
- UK GEMINI, the Geo-spatial Metadata Interoperability Initiative, is a geographic metadata standard for describing geospatial data resources.
- Other metadata standards are available in the RDA (Research Data Alliance) metadata standards catalog.
Data can be standardised by completing a pre-designed data template, such as the MEDIN biodiversity data guidelines, or by following specific guidance to format datasets according to a checklist of mandatory and conditional fields which must be completed for compliance. Data are recorded using controlled vocabularies, which have standardised definitions and term titles for pieces of information, removing any ambiguity within a dataset. Data archive centres can provide comprehensive guidance on data standardisation, and further information is available on the websites for each data standard. If a standard for the type of data the citizen science project will produce does not yet exists, data archive centres can also help to develop a suitable, tailored standard for your data.
Recommendations
- Data and metadata need to comply with standards to support FAIR data principles.
- If a data standard for your data type does not exists, contact a data archive centre to enquire about creating a new standard guideline.
Case Study
The JCDP worked with MEDIN to create a new data standard for cetacean occurrence data to maximise the value of these data through collation and promoting universal access. This aims to increase the amount of cetacean data that can be collated and published on the JCDP data portal through ensuring all data complies with the same standard. This is an example of a standard guidance document which users may use to inform their data submissions.
MEDIN also publishes data guidelines in the form of spreadsheet templates that data providers can fill in with their data. Completing these forms with all mandatory and conditional information ensures data are MEDIN.compliant. An example of the form is the MEDIN data guideline for ad-hoc sightings and non-effort based surveys of marine life, which may be used by casual observers who wish to submit ad-hoc sightings. The guideline is split into 3 general levels: the general metadata (an overview of the dataset) the detailed metadata (the methods) and the data themselves. The latter can be split into many different tabs containing information about taxa, biotopes, sampling stations, sampling events, etc.
Metadata
Metadata is information that describes data. As a minimum, metadata describes who collected the data, where they were collected, when they were collected, what the dataset(s) describes, how the dataset was collected, and a contact person/organization or data provider from which a copy of the data can be obtained. Metadata standards ensure metadata are collected in a consistent way which allows them to be shared or combined to form a national information resource and help to answer management questions both nationally and internationally. The importance of recording metadata is highlighted in this video. High-quality citizen science data will have a metadata instance which effectively describes the dataset making it as accessible as possible for any users.
Recommendations
- Metadata should be standardised and should contain all necessary information for users to understand and re-use the dataset.
Case Study
MEDIN metadata records are published on the MEDIN Portal. Each record gives metadata such as the abstract, data holder, use constraints, geographic extent, a link to the data location, unique identifiers, and survey dates. Users can search for specific survey data by finding their metadata record, and should be able to download the data within two clicks of finding the metadata record. Contact information is also available should the users require additional information.
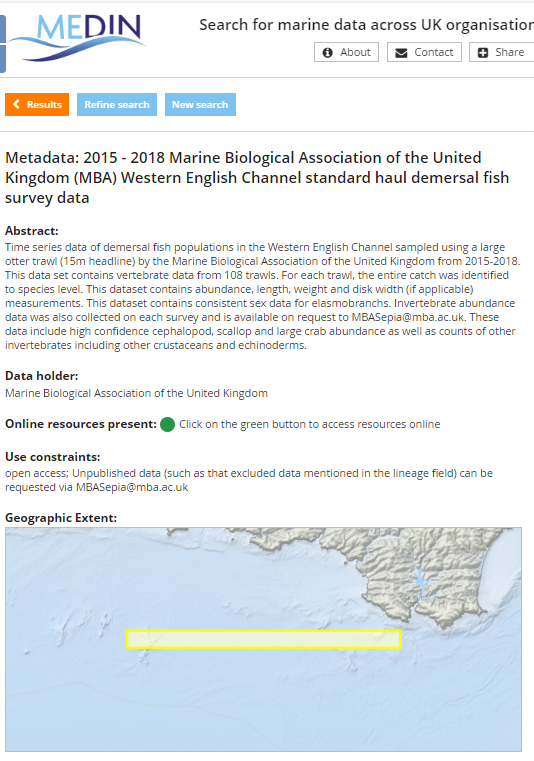
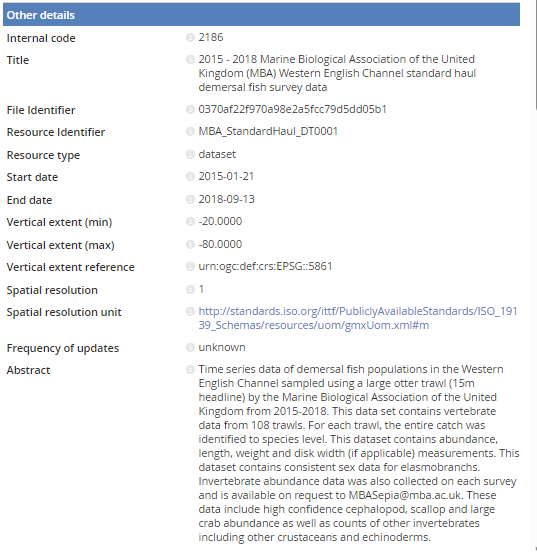
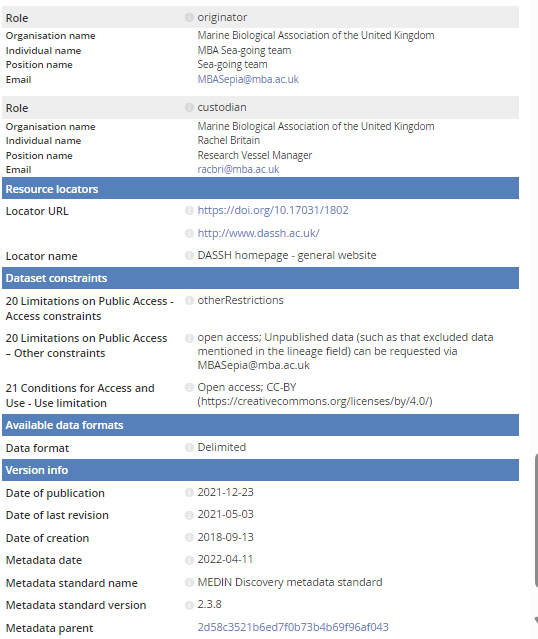
Controlled vocabularies
Controlled vocabularies are one of the tools used to make datasets more consistent and standardised, supporting FAIR principles by making data interoperable and reusable. They make potentially ambiguous terms explicit and clearly defined which reduces the risk of misinterpretation. For example, the common name for a species may be different in different areas, so by using a controlled vocabulary for taxon name with a standard species name and code it is clear which species the data is describing. They also allow the online publication of data in a format that is machine readable, which means that APIs and machine learning can collate data from different sources which can be used to conduct analyses.
Controlled vocabularies are used in metadata standards in fields like keywords, geographic coverage terms, formats of data files, and in data standards to describe fields such as categories and models of instrument, organisations and research vessels. Some data standards, such as the MEDIN guidelines, specify which controlled vocabularies to use in specific data fields, but data archive centres (DAC) can provide additional assistance with standardising data with controlled vocabularies.
Commonly used vocabularies in biodiversity data standards are:
- WoRMS, a catalogue of marine species that includes unique “aphiaID” codes for each taxon name
- MSBIAS, a subset of WoRMS for the British Isles and adjacent seas.
- ICES reference codes for vessel names (C17), sampling devices categories (L05), sampling devices (L22).
- Vocabularies for biological features such as size (S09), sex (S10), development stage (S11), morphology (S14), colour (S15)
- EPSG codes for coordinate reference systems
- P02 vocabulary for metadata keywords
- EUNIS codes for biotopes: M21, M20 and M24
- EDMO organisation codes e.g. for analytical laboratories and data owners
There are vocabulary search clients such as the BODC client and SeaDataNet client in which users can search across many different vocabularies in the NERC vocabulary server. The OBIS Manual has specific guidance on how to select a suitable term in controlled vocabularies, and how to map terms to specific standards such as Darwin Core (DwC), and MEDIN also provides further guidance on the use of controlled vocabularies. Please note that this is not a comprehensive list of controlled vocabularies.
Recommendations
- Use controlled vocabularies when standardising data to ensure data is unambiguous and interoperable.
- Contact the relevant DAC for advice on which controlled vocabularies to use for a specific field in a dataset.
Data processing tools
In order to standardise data and quality assure data, tools may be required to conduct automated checks or to convert data. Projects that produce high quality data will provide any volunteers or staff that will process data with the adequate training to ensure they are competent with the tool and to reduce the risk of mistakes. For example, if transcribing data from one spreadsheet to another, there is a risk of copying errors due to Microsoft Excel’s automatic formatting. This highlights the importance of conducting a thorough quality assurance following the standardisation and processing of data into final versions. The tools themselves can also be used to check that the processing was carried out accurately, and to check for transcription or recording errors. Examples of data processing tools, in addition to Excel formulae, include Python, R packages, QGIS, and coordinate conversion tools such as Grid Reference Finder or OBIS's batch coordinate converter.
Recommendations
- Ensure any volunteers or staff that process data are skilled in the processing tools to reduce the risk of errors.
Case Study
The WoRMS or MSBIAS taxon match tools can be used to standardise multiple taxon names at once. These tools take a list of scientific names, which must be supplied in a spreadsheet or a plain text file containing only the scientific names, and compares them to the catalogue of taxon names to match them with each unique reference for each species, known as the aphiaID. A user can select other parameters to display, for example the full classification of the taxon, and can download this as a spreadsheet. The output of the taxon match tools will provide accepted taxon names, so if nomenclature changes, the output will reflect this. This information can be used within the dataset, or as a way to check existing names are correct and accepted.
Quality Assurance (QA)
Following data collection and standardisation, the data should be quality assured to correct any errors that may have arisen during the recording of data or during the conversion to the standardised format. Citizen scientists may have varying levels of data and computer literacy, so errors introduced by copying values between Excel spreadsheets, converting units, and selecting unique identifiers for controlled vocabularies may occur. A thorough quality assurance procedure will increase the reliability of data, and by checking adherence to standards it will also increase the interoperability and reusability.
When submitting standardised data to a data archive centre such as DASSH, it will be quality assured by experienced Data Officers, cross checking all information, images and videos, against the original data and relevant standards, reviewed to assess data quality. Any changes made are recorded to enable traceability of modifications. If any information is missing to comply with data standards, the DAC will then contact the data provider to obtain this and clarify any ambiguities. Further information on DASSH quality assurance procedures are available here.
There are many tools that can be used to assist with the quality assurance of a dataset including:
- WoRMS (World Register of Marine Species) taxon match tool. Checks taxon names are accepted and provides the correct aphia IDs, which are unique and standardised numerical codes for each name.
- MSBIAS (Marine Species of the British Isles and Adjacent Seas) taxon match tool. The same as above but only for marine species in the British Isles.
- Tools used to validate datasets for sharing to OBIS are available in the OBIS manual.
- QGIS to check coordinates of data points are accurate according to metadata and the geographical range of species recorded.
- Grid reference finder to check conversions of coordinates from one format e.g. British National Grid, to another e.g. decimal degrees.
- Google Earth can be used to check coordinates and localities of sampling events, particularly when checking against named locations.
- NBN Atlas can be used to check current distributions of species in the UK.
Recommendations
- A thorough quality assurance of the data should be conducted to check for processing errors, controlled vocabularies and standardisation to increase data reliability
- Liaise with the DAC that will archive and publish the data from the project to see what their quality assurance procedure and what information they require
Case Study
Citizen science projects such as Seasearch conduct internal quality assurance for their recording forms. In this case, the process consists of an initial validation conducted locally or by the national coordinator, data entry into the Marine Recorder database by experienced personnel, merging and final checks carried out by the National Coordinator and Seasearch Data Officer, and ongoing data management where necessary after the data has been published.
Validation
Validation of data against the selected standards ensures that the data are compliant with those standards to enable onward publication and interoperability of the data. While quality assurance checks for general data errors, validation specifically checks for standard compliance prior publication of the data. Validation may be carried out as part of the QA step, or it can be treated as a separate step before publication. Examples of validation procedures include:
- DASSH's MEDIN Guideline Validation tool. This tool checks that a dataset which has been standardised into the MEDIN guideline format is compliant, flagging any issues or fields which do not match the standards.
- GBIF data validator. This provides a report on the syntactical correctness and validity of the dataset, requires the dataset to be submitted in Darwin Core (DwC) format.
- MEDIN Discovery Metadata validation (Schematron). When completing a MEDIN metadata record using the online editor, the editor will run the record through a validation before it can be exported to the MEDIN Discovery Metadata Portal. The metadata records can also be exported as XML files, or can be created using Maestro, a tool which saves the records as XML files. The schematron can be used with the XML Schema Definition to validate XML metadata records.
Recommendations
- Once quality assured, the data should be validated against the selected data standard.
Summary & Checklist
Following data collection and prior to data archival and publication, data will need to be processed to ensure it is standardised, verified and quality assured to increase data quality. The following points should be taken into consideration:
- Ensure records are verified prior to further processing to increase data accuracy and reliability
- Data and metadata need to comply with standards to support FAIR data principles
- If a data standard for your data type does not exists, contact a data archive centre to enquire about creating a new standard guideline
- Metadata should be standardised and should contain all necessary information for users to understand and re-use the dataset
- Use controlled vocabularies when standardising data to ensure data is unambiguous and interoperable
- Contact the relevant DAC for advice on which controlled vocabularies to use for a specific field in a dataset
- Ensure any volunteers or staff that process data are skilled in the processing tools to reduce the risk of errors
- A thorough quality assurance of the data should be conducted to check for processing errors, controlled vocabularies and standardisation to increase data reliability
- Liaise with the DAC that will archive and publish the data from the project to see what their quality assurance procedure and what information they require
- Once quality assured, the data should be validated against the selected data standard.
- Contact the relevant DAC for advice about data standardisation and quality assurance
- Provide the DAC with all required information to conduct a thorough QA if they are to do this step
Preserve
Overview
The archiving of data created by a projects preserves its longevity beyond the lifespan of the project. Preservation of data can be categorised as short-term or long-term2.
Short-term preservation may involve backing up data whilst data processing is carried out, often storing data and raw files on physical devices such as hard drives, as paper records or scanned versions of these, on USBs, DVDs or online e.g. on a Cloud drive. Storing data on physical devices risks the loss of these data through damage or loss of them, and has limitations with storage capacity, and many agencies actively discourage this form of data storage. With modern data types such as imagery, acoustics and genetic data, files can be very large so cloud storage provides more flexibility with storage capacity.
Long-term storage of citizen science data should be done via data repository or archive centre, which benefit from effective data management, reduces the risk of losing physical copies of data, have the capability to archive large datasets consisting of multiple data types. This preserves the data so it can be accessed by a wide range of future users3.
2 Shwe, K. M. (2020). Study on the Data Management of Citizen Science: From the Data Life Cycle Perspective. Data and Information Management, 279-296. doi:https://doi.org/10.2478/dim-2020-0019
3 Rambonnet, L., Vink, S. C., Land-Zandstra, A. M. and Bosker, T. (2019). Making citizen science count: Best practices and challenges of citizen science projects on plastics in aquatic environments. Marine Pollution Bulletin, 145, 271-277. doi:https://doi.org/10.1016/j.marpolbul.2019.05.056
Activities
Data archiving
Data produced by a citizen science project may be digital or physical. Digital data could be spreadsheets, digital reports, databases, acoustics, digital images and GIS files. They can be supplied to a data archive centre in this format for their archival as raw files, along with standardised versions of dataset and metadata that can be published to make them accessible. Some DACs such as DASSH may be able to archive novel data types such as eDNA data and spatial polygon data, further information can be found by contacting the relevant DAC,
Physical data such as survey sheets, record cards, photographs, notebooks, reports will need to be digitised prior to archiving if the data are to be published online. Although it is possible to archive these physical assets in repositories, museums and data archive centres as they are, by creating a digital record of them they become discoverable and usable by a much wider range of online users and organisations, supporting FAIR data principles and maximising the impact of these data. The relevant DAC should be contacted to establish what their capacity is to store physical data. If they do not support physical data they may be able to advise on alternative archiving solutions, for example with museums or libraries.
The archiving of these data with a data archive centre is often a stipulation of funding grants, so it is highly recommended that this step is carried out promptly after the creation and processing of the data. It also ensures the longevity of the data, especially in cases where projects conclude, where organisation that own the data dissolve, or where individuals who own the data are no longer around.
Recommendations
- Archive both the raw and processed data with a DAC.
- Digitise any physical data and archive to maximise accessibility, and to protect data against physical damage and loss.
Case Study
Kew Royal Botanical Gardens have launched a digitisation project which aims to digitise its entire collection of more than 8 million plant and fungal specimens, making them openly accessible on a global online platform. Many of those working on the project are volunteers working to preserve the data for these specimens.
Data publishing
When citizen science data are published and made openly available for re-use, they will have a greater impact as they’re more widely available for use in research and data-driven policy. This impact is maximised when data are FAIR because they’re published in a findable location like a data portal, under an open-access license, are standardised and well described with standardised metadata, and are in a format that can be reused and understood clearly. Similar to archiving, the publishing of data produced by a project funded by grants is often a mandatory requirement at the end of the project.
Recommendations
- Publish data under an open access license to maximise its impact and longevity.
Data Archive Centres (DACs)
Data archive centres fulfil the vital role of preserving data by archiving and publishing it. High quality data collected for UK marine assessments should be archived at a nationally or internationally accredited data centre to ensure long-term access to these data, e.g. MEDIN Data Archive Centre, ICES, other data centre accredited with Core Trust Seal.
Recommendations
- Archive data with an accredited data archive centre to ensure long-term access.
Summary & Checklist
- Archive both the raw and processed data with a DAC
- Digitise any physical data and archive to maximise accessibility, and to protect data against physical damage and loss
- Publish data under an open access license to maximise its impact and longevity
- Archive data with an accredited data archive centre to ensure long-term access
Re-use
Overview
Making data FAIR and as openly accessible as possible enables users to easily find data and re-use it for purposes such as research, conservation, environmental monitoring, and policy. Data users may want to access citizen science data to inform the planning of future citizen science projects, or they be citizen scientists who wish to see where the data has been published and use it in analyses.
Activities
Finding data
Once data are published, they can be made available on data portals, which enable the metadata and datasets to be findable and accessible. There are national and international portals, some of which are portals maintained by the data archive centres themselves. Examples of locations to find data, including those produced by citizen scientists, are:
- MEDIN Discovery Metadata Portal. A collection of metadata records for UK datasets archived by MEDIN DAC.. Users can search for metadata by title, unique identifier (GUID) dates, regions, season of interest, or specific controlled vocabulary parameters. The resulting metadata records will contain unique identifiers, contact details for data providers, download links, and general metadata about the dataset.
- DASSH Mapper. This shows all individual species records held in the DASSH database from standardised marine biodiversity datasets within the UK submitted by data providers. Users may search for specific taxon names, survey name, biotope, originator, owner, and they can filter for eDNA-derived records. Search results are shown in the map, and as a list further down the page where each survey should contain a link to the metadata for the survey from which the records was obtained.
- NBN Atlas. An online tool for accessing publicly available biodiversity data from the UK. Users can search for taxa or surveys and the results can be viewed as records on a map, or as charts summarising information. Metadata are given for these data, with links to the original datasets available.
- GBIF. International network and data infrastructure funded by the world's governments and aimed at providing anyone, anywhere, open access to data about all types of life on Earth. Similar to NBN Atlas, when searching for records the results can be visualised in a range of different ways, and the metadata and reference to the original dataset are also provided.
- OBIS. Global open-access data and information clearing-house on marine biodiversity for science, conservation and sustainable development. Data can be searched for and viewed on the mapper by filtering the results and saving the filters as layers. These layers of data can then be downloaded. The OBIS API can also be used to query the OBIS database to return specific information.
- EMODnet. Portals for data products in Europe are divided into 7 themes: Bathymetry, Biology, Chemistry, Geology, Human Activities, Physics and Seabed habitats.
- EurOBIS. Publishes distribution data on marine species, collected within European marine waters or collected by European researchers outside European marine waters. These data are also available on the EMODnet portal, and on a regular basis, all the EurOBIS data are sent to OBIS, which in turn sends its data to GBIF.
- Marine Recorder. Data from Marine Recorder can be viewed as a downloadable snapshot in the format of an Microsoft Access database.
Recommendations
- Make use of data portals, platforms and open access databases to find data produced by citizen science, and to find data to inform the planning of new citizen science projects.
Metadata
Metadata is essential for data re-use, as it provides important information about their provenance i.e. the materials and methods of data collection and any processing conducted and conditions for re-use. The metadata also prevents misinterpretation of data through the use of controlled vocabularies with explicit definitions.
Recommendations
Use the metadata to determine re-use conditions and to obtain information to allow accurate interpretation of the data.
Licensing and permissions for data re-use
When using published data for further analyses, to inform research, reports, conservation and creating new publications from the data, the data license terms and re-use conditions must be followed, for example by crediting data owners, and not using these data for commercial purposes. Commonly used data licenses are:
- Open Government License (OGL). This license allows you to:
- Copy, publish, distribute and transmit the data
- Adapt the information
- Exploit the data commercially and non-commercially
However, you must: - Acknowledge the data source and include any attribution statement specified by the Data Provider(s) and, where possible, provide a link to the license information.
- More information on this license can be found on the National Archives website.
- Creative Commons No rights reserved license (CC0). Under this license, the data provider has waived any rights to the data under copyright or Intellectual Property Rights law. This license allows you to:
- Copy, modify and distribute the data, including for commercial purposes, without seeking permission from the data provider
- More information on CC0 can be found on the Creative Commons website
- Creative Commons license with attribution (CC-BY). This license allows you to:
- Share – copy and redistribute the data in any medium or format
- Adapt – remix, transform and build upon the data
- Data can be used for any purpose, including commercial use
You must: - Give appropriate credit to the data provider
- Provide a link to the license
- Indicate what, if any, changes have been made
- More information on this license can be found on the Creative Commons website
- Creative Commons with attribution non-commercial (CC-BY-NC). Data under this license can be used under the same conditions as the CC-BY license except the data cannot be used for commercial purposes. More information on this license can be found on the Creative Commons website.
Recommendations
- Follow the license restrictions and re-use conditions for the data used.
Sustainability
Re-using data to inform new projects, and to determine where gaps in existing data are, can make a project more sustainable as it can prevent the duplication of data collection events, it can save time that may be otherwise spent organising and running new data collection events, and can improve the design of a project if it is well-informed by existing data. By following FAIR principles and making data openly accessible to be reused, there can be further financial and environmental benefits. These benefits include:
- Time saved by reducing time spent searching for existing marine data, as it should be easily and openly accessible. There is also a time saving benefit to organisations as their own data will be managed efficiently via a data archive centre.
- Prevention of duplication of primary data gathering and research efforts as there is access to data collected by others.
- Improved decision-making due to greater availability of data, as more existing data can be gathered, and better primary data can be collected.
Recommendations
- Where possible, re-use existing data in order avoid unnecessary duplication of data collection efforts and to inform new citizen science projects.
Case Study
The MEDIN Cost Benefit Analysis report calculated a net benefit of £52.4 million over a 10-year period, from the services that MEDIN provides to its users. These include the accreditation of data archive centres, the MEDIN Portal for finding UK marine data, and a metadata standard to provide information about datasets.
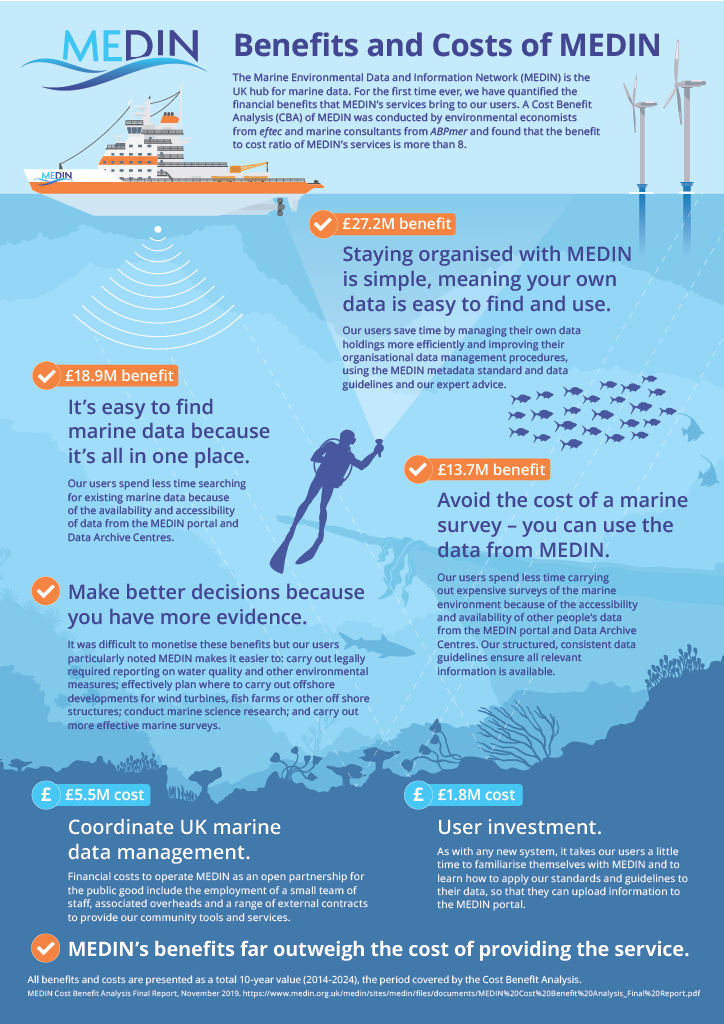
Summary & Checklist
When re-using data, the key elements to consider are:
- Make use of data portals, platforms and open access databases to find data produced by citizen science, and to find data to inform the planning of new citizen science projects
- Use the metadata to determine re-use conditions and to obtain information to allow accurate interpretation of the data
- Follow the license restrictions and re-use conditions for the data used
- Where possible, re-use existing data in order avoid unnecessary duplication of data collection efforts and to inform new citizen science projects
Checklist
Plan
Activity Recommendations
Data collection protocols
- Select a standard data collection protocol, if it exists, or if new protocols are developed, aim to follow exisiting standards, and outline this in the data management plan
- Determine the likely and required spatial coverage of the project
Data collection tools
- Plan which tools are required, accounting for any training required for staff and/or volunteers to use them.
- Reflect on cost, accuracy, precision, and suitability to the environment.
Licensing and permissions for data collection
- Plan and seek any permissions that may be required for the task, including access permissions from landowners, consent from participants to use the collected data and media such as photographs for analyses, research and publication and whether these can be used for social media and advertising purposes.
- Plan the creation of permission forms for the use and storage of personal data to comply with GDPR regulations, keeping this to only data that is necessary.
Verification and quality control (QC) schemes
- Outline any on-site and post-collection data verification methods and data quality control (QC) schemes in the data management plan
Training
- Outline training in the data management plan, considering complexity of data collection protocols, equipment used, experience level of participants and the requirement for standardisation of training materials.
Data standardisation
- Identify appropriate data standards for the data being collected and determine which mandatory information needs to be recorded during the data collection event. If data are to be submitted to a MEDIN DAC, choose the appropriate MEDIN data guideline.
Metadata
- Research metadata standards in the planning stage to ensure all mandatory information is collected in the data collection and processing stages.
Data processing tools
- Define data processing tools in the data management plan to allocate appropriate training and resources.
Quality Assurance (QA)
- Outline the quality assurance procedures in the data management plan to determine which data need to be retained for effective data QA.
- Contact DAC to establish whether they have their own quality assurance procedure and if so, what information they require to conduct a QA of the submitted data.
Data Archive Centres (DACs)
- Specify the data archive centre you will archive and publish data through in the data management plan.
- Contact the data archive centre at an early stage to receive specific guidance relevant to your data.
Data flow pathway
- Determine the route the data will take once collected, considering whether they will be submitted as individual records or whole datasets.
- Plan to communicate the data pathway clearly with citizen scientists to increase future engagement.
Licensing and permissions for data re-use
- Outline any conditions to data sharing, access and reuse the data produced by the citizen science project will have in the data management plan.
Maintainance
- Plan how data resulting from the project will be maintained and by who, should the project end, corrections be required, or additional data be added at a later stage.
Checklist
A citizen science project that produces high quality, FAIR data, should cover the following points in the data management plan:
- Select a standard data collection protocol, if it exists, or if new protocols are developed, aim to follow exisiting standards, and outline this in the data management plan
- Determine the likely and required spatial coverage of the project
- Plan which tools are required, accounting for any training required for staff and/or volunteers to use them
- Reflect on cost, accuracy, precision, and suitability to the environment
- Plan and seek any permissions that may be required for the task, including access permissions from landowners, consent from participants to use the collected data and media such as photographs for analyses, research and publication and whether these can be used for social media and advertising purposes
- Plan the creation of permission forms for the use and storage of personal data to comply with GDPR regulations, keeping this to only data that is necessary
- Outline any on-site and post-collection data verification methods and data quality control (QC schemes in the data management plan
- Outline training protocols, considering complexity of data collection protocols, equipment used, experience level of participants and the requirement for standardisation of training materials
- Identify appropriate data standards for the data being collected and determine which mandatory information needs to be recorded during the data collection event. If data are to be submitted to a MEDIN DAC, choose the appropriate MEDIN data guideline
- Research metadata standards in the planning stage to ensure all mandatory information is collected in the data collection and processing stages
- Define data processing tools in order to allocate appropriate training and resources
- Outline the quality assurance procedures to determine which data need to be retained for effective data QA
- Contact DAC to establish whether they have their own quality assurance procedure and if so, what information they require to conduct a QA of the submitted data
- Specify the data archive centre you will archive and publish data with
- Contact the data archive centre at an early stage to receive specific guidance relevant to your data
- Determine the route the data will take once collected, considering whether they will be submitted as individual records or whole datasets
- Plan to communicate the data pathway clearly with citizen scientists to increase future engagement
- Outline any conditions to data sharing, access and reuse the data produced by the citizen science project will have
- Plan how data resulting from the project will be maintained and by who, should the project end, corrections be required, or additional data be added at a later stage
Collect
Activity Recommendations
Data collection tools
- Select tools which are standardised and calibrated across users, and suitable to volunteer skills and the environment in which they’ll be used
- Test tools at the survey location prior to the collection event to determine potential issues and errors
- Tailor the tools used to the type of data that will be collected and the scope of the project
Data collection protocols
- Select standardised data collection protocols which are tested by professionals prior to being carried out by volunteers.
- Allow adequate time for training and testing the protocols alongside data collection.
Verification and quality control (QC) schemes
- Have a data verification or quality control procedure in place to check records for accuracy at the data collection event.
Licensing and permissions for data collection
- Ensure volunteers have signed relevant data use permission forms or selected licenses for their sightings if submitting data individually.
- Communicate restrictions resulting from obtaining permission to access specific survey sites to all volunteers.
Training
- During data collection, ensure all volunteers have received appropriate training to carry out the work required, considering experience, tasks and tools required, and difficulty.
Checklist
When running a citizen science data collection event, such as a biodiversity survey, the following should be considered:
- Select tools which are standardised and calibrated across users, and suitable to volunteer skills and the environment in which they’ll be used
- Test tools at the survey location prior to the collection event to determine potential issues and errors
- Tailor the tools used to the type of data that will be collected and the scope of the project
- Select standardised data collection protocols which are tested by professionals prior to being carried out by volunteers
- Allow adequate time for training and testing the protocols alongside the data collection
- Have a data verification or quality control procedure in place to check records for accuracy at the data collection event
- Ensure volunteers have signed relevant data use permission forms or selected licenses for their sightings if submitting data individually
- Communicate restrictions resulting from obtaining permission to access specific survey sites to all volunteers
- Ensure all volunteers have received appropriate training to carry out the work required, considering experience, tasks and tools required, and their difficulty
Process
Activity Recommendations
Data Archive Centres (DACs)
- Contact the relevant DAC for advice about data standardisation and quality assurance.
- Provide the DAC with all required information to conduct a thorough QA if they are to do this step.
Data verification
- Ensure records are verified prior to further processing to increase data accuracy and reliability.
Data standardisation
- Data and metadata need to comply with standards to support FAIR data principles.
- If a data standard for your data type does not exists, contact a data archive centre to enquire about creating a new standard guideline.
Metadata
- Metadata should be standardised and should contain all necessary information for users to understand and re-use the dataset.
Controlled vocabularies
- Use controlled vocabularies when standardising data to ensure data is unambiguous and interoperable.
- Contact the relevant DAC for advice on which controlled vocabularies to use for a specific field in a dataset.
Data processing tools
- Ensure any volunteers or staff that process data are skilled in the processing tools to reduce the risk of errors.
Quality Assurance (QA)
- A thorough quality assurance of the data should be conducted to check for processing errors, controlled vocabularies and standardisation to increase data reliability
- Liaise with the DAC that will archive and publish the data from the project to see what their quality assurance procedure and what information they require
Validation
- Once quality assured, the data should be validated against the selected data standard.
Checklist
Following data collection and prior to data archival and publication, data will need to be processed to ensure it is standardised, verified and quality assured to increase data quality. The following points should be taken into consideration:
- Ensure records are verified prior to further processing to increase data accuracy and reliability
- Data and metadata need to comply with standards to support FAIR data principles
- If a data standard for your data type does not exists, contact a data archive centre to enquire about creating a new standard guideline
- Metadata should be standardised and should contain all necessary information for users to understand and re-use the dataset
- Use controlled vocabularies when standardising data to ensure data is unambiguous and interoperable
- Contact the relevant DAC for advice on which controlled vocabularies to use for a specific field in a dataset
- Ensure any volunteers or staff that process data are skilled in the processing tools to reduce the risk of errors
- A thorough quality assurance of the data should be conducted to check for processing errors, controlled vocabularies and standardisation to increase data reliability
- Liaise with the DAC that will archive and publish the data from the project to see what their quality assurance procedure and what information they require
- Once quality assured, the data should be validated against the selected data standard.
- Contact the relevant DAC for advice about data standardisation and quality assurance
- Provide the DAC with all required information to conduct a thorough QA if they are to do this step
Preserve
Activity Recommendations
Data archiving
- Archive both the raw and processed data with a DAC.
- Digitise any physical data and archive to maximise accessibility, and to protect data against physical damage and loss.
Data publishing
- Publish data under an open access license to maximise its impact and longevity.
Data Archive Centres (DACs)
- Archive data with an accredited data archive centre to ensure long-term access.
Checklist
- Archive both the raw and processed data with a DAC
- Digitise any physical data and archive to maximise accessibility, and to protect data against physical damage and loss
- Publish data under an open access license to maximise its impact and longevity
- Archive data with an accredited data archive centre to ensure long-term access
Share
Activity Recommendations
Data publishing
- Following data archival, data must be published to enable users to find and use it, either with a DAC or via a data submission portal
Data Archive Centres (DACs)
- Contact the relevant DAC to find out about their data publication procedure.
Data flow pathway
- Become familiar with the data flow resulting from your choice of publication platform, i.e. DAC or record submission portal.
Licensing and permissions for data re-use
- Select a license or explicit terms of use for the published citizen science data
- Choose a license which is as open as possible to enable open access and maximise reuse
Social media
- Share news of new data updates and publications via social media to increase data discoverability.
Checklist
- Following data archival, data must be published to enable users to find and use it, either with a DAC or via a data submission portal
- Contact the relevant DAC to find out about their data publication procedure.
- Become familiar with the data flow resulting from your choice of publication platform, i.e. DAC or record submission portal
- Select a license or explicit terms of use for the published citizen science data
- Choose a license which is as open as possible to enable open access and maximise reuse
- Share news of new data updates and publications via social media to increase data discoverability
Re-use
Activity Recommendations
Finding data
- Make use of data portals, platforms and open access databases to find data produced by citizen science, and to find data to inform the planning of new citizen science projects.
Metadata
Use the metadata to determine re-use conditions and to obtain information to allow accurate interpretation of the data.
Licensing and permissions for data re-use
- Follow the license restrictions and re-use conditions for the data used.
Sustainability
- Where possible, re-use existing data in order avoid unnecessary duplication of data collection efforts and to inform new citizen science projects.
Checklist
When re-using data, the key elements to consider are:
- Make use of data portals, platforms and open access databases to find data produced by citizen science, and to find data to inform the planning of new citizen science projects
- Use the metadata to determine re-use conditions and to obtain information to allow accurate interpretation of the data
- Follow the license restrictions and re-use conditions for the data used
- Where possible, re-use existing data in order avoid unnecessary duplication of data collection efforts and to inform new citizen science projects
Glossary
ABCD | Access to Biological Collection Data. A comprehensive standard for the access to and exchange of data about specimens and observations. |
ADS | Archaeology Data Service. A digital repository for UK archaeology and historic environment daata, and a MEDIN historic environment DAC. |
API | Application Programming Interface. A set of rules or protocols that let software applications communicate with each other to exchange data, features and functionality. |
BGS | British Geological Survey. Geological survey and global geoscience organisation that provides geoscientific data, information and knowledge, and is a MEDIN geological DAC. |
Bioblitz | A communal citizen-science effort to record as many species within a designated location and time period as possible. |
BioCASe | Biological Collection Access Service. A transnational network of primary biodiversity repositories. It links together specimen data from natural history collections, botanical/zoological gardens and research institutions worldwide with information from huge observation databases. |
BODC | British Oceanographic Data Centre. MEDIN Oceanography DAC that looks after and distributes data concerning the marine environment. |
CC-BY | Creative Commons license where credit must be given to the creator. |
CC-BY-NC | Creative Commons license where credit must be given to the creator and only non-commercial uses are permitted. |
CC-BY-SA | Creative Commons license where credit must be given to the creator and adaptations must be shared under the same terms. |
CC0 | Creative Commons No Rights Reserved license. Enables creators and owners of copyright- or database-protected content to waive those interests in their works and place them as completely as possible in the public domain. |
Cefas | Centre for Environment, Fisheries and Aquaculture Science. UK government's marine and freshwater science experts that provide data and advice to government and overseas partners, and MEDIN fisheries DAC. |
DAC | Data Archive Centre. A collection of data archive centres work with different data themes as part of the Marine Environmental Data and Information Network. |
DASSH | The Archive for Marine Species and Habitats Data, based at the Marine Biological Association in the UK. |
DOI | Digital Object Identifier. A digital identifier of an object - physical, digital, or abstract. |
DwC | Darwin Core data standard that facilitates the sharing of information about biological diversity by providing identifiers, labels and definitions. Primarily based on taxa and their occurrence in nature, and uses the file format DwC-A. |
DwC-A | Darwin Core Archive standard file format used in the Darwin Core standard. |
EDMO | European Directory of Marine Organisations. Contains up-to-date addresses and activity profiles of research institutes, data holding centres, monitoring agencies, governmental and private organisations, that are in one way or another engaged in oceanographic and marine research activities, data & information management and/or data acquisition activities. |
EML | Ecological Metadata Language. A metadata standard for ecological metadata. |
EMODnet | European Marine Observation and Data Network. A network of organisations supported by the EU's integrated maritime policy that work together to observe the sea, process the data according to international standards and make that information freely available as interoperale data layers and data products. |
EPSG | European Petroleum Survey Group. The EPSG Geodetic Parameter Dataset contains definitions of coordinate reference systems and coordinate transformations which may be global, regional, national or local in application. It is maintained by the Geodesy Subcommittee of the IOGP Geomatics Committee. |
EUNIS | The European Nature Information System. Contains a controlled vocabulary to describe habitat types. |
EurOBIS | European Ocean Biodiversity Information System. Publishes data on marine species, collected within European marine waters or collected by European researchers outside European marine waters. |
FAIR | Findable Accessible Interoperable Reusable. The guiding principles for scientific data management and stewardship. |
GBIF | Global Biodiversity Information Facility |
GDPR | General Data Protection Regulation. |
GIS | Geographic Information Systems |
GUID | Globally Unique Identifier |
HES | Historic Environment Scotland. Scottish data archive centre and MEDIN historic environment DAC. |
ICES | International Council for the Exploration of the Seas. Marine science organisation that provides evidence on the state and sustainable use of the seas and oceans. |
iNaturalist | A global community for nature lovers where you can record your own nature observations and get help identifying them. iNaturalistUK is a member of the iNaturalist network and is co-ordinated in the UK by the National Biodiversity Network Trust (NBN Trust) with the support of the Marine Biological Association (MBA) and the Biological Records Centre (BRC). |
INSPIRE | Infrastructure for Spatial Information in the European Community. It places legal obligations on public bodies to publish particular datasets that are geo-spatial, in any of the 34 INSPIRE themes and should be existing data. |
IPT | Integrated Publishing Toolkit. A free open-source software developed by GBIF and used by organisations around the world to create and manage repositories for sharing biodiversity datasets. |
iRecord | Recording platform for UK wildlife |
JCDP | The Joint Cetacean Data Programme promotes and facilitates cetacean data standardisation and maximises value thorugh collation and the enabling of universal access to these data. |
JNCC | Joint Nature Conservation Committee. |
LifeWatch ERIC | A European Research Infrastructure Consortium providing e-Science research facilities to scientists investigating biodiversity and ecosystem functions and services in order to support society in addressing key planetary challenges. |
Maestro | Software for creating MEDIN-compliant metadata records. |
Marine Recorder | A benthic survey data management system used widely within the UK’s statutory nature conservation bodies to store and query benthic sample data across the UK’s offshore and inshore waters. The system is able to store species occurrence data (with associated measurements), biotope information in the Marine Habitat Classification for Britain & Ireland and physical attribute data. The system maintains consistency and relationships between sample information, measurements and surveys allowing for accessible querying of the database. |
Marine Scotland | Marine Scotland is part of the Scottish government, and a MEDIN fisheries DAC, responsible for managing Scotland's marine and freshwater environment. |
MarLIN | The Marine Life Information Network. Provides information on the biology of speices and the ecology of habitats found around the coasts and seas of the British Isles. |
MBA | The Marine Biological Association of the United Kingdom. A learned society with a scientific laboratory that undertakes research in marine biology. |
MEDIN | Marine Environmental Data and Information Network. |
Met Office | The MEDIN data archive centre for marine meteorological (metocean) data in the UK. |
MMO | Marine Management Organisation, an executive non-departmental public body, sponsored by the Department for Environment, Food and Rural Affairs. Find out more |
mNCEA | Marine Natural Capital and Ecosystem Assessment Programme. A research and development program funded by Defra. |
MSBIAS | Marine Species of the British Isles and Adjacent Seas. Register of marine species, which is a subset of WoRMS containing species found in the British Isles and Adjascent Seas. |
NBN | National Biodiversity Network in the UK |
NBN Atlas | NBN data repository |
NCEA | Natural Capital and Ecosystem Assessment. A science innovation and transformation programme, which spans across land and water environments. It has been set up to collect data on the extent, condition and change over time of England’s ecosystems and natural capital, and the benefits to society. |
NERC | Natural Environment Research Council. |
NMBAQC | The NE Atlantic Marine Biological Analytical Quality Control Scheme provides a source of external Quality Assurance (QA) for laboratories engaged in the production of marine biological data. |
OBIS | Ocean Biodiversity Information System. A global open-access data and information clearing-house on marine biodiversity for science, conservation and sustainable development. |
OGL | Open Government License for public sector information. |
ORCA | Marine conservation charity dedicated to the long-term study and protection of whales, dolphins and porpoises and their habitats around the world. |
Python | Python is an interpreted, object-oriented, high-level programming language with dynamic semantics. The Python interpreter and the extensive standard library are available in source or binary form without charge for all major platforms, and can be freely distributed. |
QA | Quality Assurance. A quality check carried out prior to publication of data to ensure the data are correct and accurate, which may include a step of data validation to check they meet the relevant data standards. |
QC | Quality Control. A quality check to ensure the data protocols and techniques produce high quality data; this may include analysing preliminary data prior to a collection event to make adjustments for elements like bias or systematic errors, and verifying records are correctly recorded prior to submission to a data archive centre which may conduct further quality assurance prior to archiving and publishing the data. |
QGIS | Quantum Geographic Information System. An open-source software used to visualise, manage, edit, analyse data and compose printable maps. |
R package | A free software environment for statistical computing and graphics. It compiles and runs on a wide variety of UNIX platforms, Windows and MacOS. |
RCAHMW | Royal Commission on the Ancient and Historical Monuments of Wales. MEDIN Historic Environment DAC which has a role in developing and promoting understanding of the archaeological, built and maritime heritage of Wales, as the originator, curator and supplier of authoritative information for individual, corporate and governmental decision makers, researchers, and the general public. |
RDA | Research Data Alliance. An international volunteer member-based organisation aiming to interact with researchers and innovators to exchange inforamtion in compliance with RDA's guiding principles. |
Repository | Data repositories are a centralised place to hold data, share data publicly, and organise data in a logical manner |
Schematron | Tool for validating MEDIN metadata records. |
SeaDataNet | Pan-European Infrastructure for Ocean and Marine Data Management. SeaDataNet has federated open digital repositories to manage, access and share data, information, products and knowledge originating from oceanographic fleets, new automatic observation systems and space sensors. |
SeaSearch | A project for recreational divers and snorkellers who want to collect information about habitats, plants and anumals they see underwater. |
The Rock Pool Project | A not-for-profit community interest company, passionate about connecting people of all ages and backgrounds to the incredible wildlife found around the UK coastline. |
UK GEMINI | UK geographic metadata standard that provides guidance on how to publish geographic metadata in a way that conforms to UK government guidelines and the relevant ISO standards. |
UKDMOS | The United Kingdom Directory of Marine Observing Systems. |
UKHO | UK Hydrographic Office. A UK executive agency and MEDIN data archive centre for hydrography, specialising in marine geospatial data. |
Validation | This determines whether a particular data point is correct and in the required format, for example data can be validated automatically using a tool that checks data are in the correct format to conform to a data standard.
|
Verification | A step to verify the accuracy, consistency and truth of the data, often involving experts confirming the accuracy of records. |
WoRMS | World Register of Marine Species. The aim of a World Register of Marine Species (WoRMS) is to provide an authoritative and comprehensive list of names of marine organisms, including information on synonymy. While the highest priority goes to valid names, other names in use are included so that this register can serve as a guide to interpret taxonomic literature. |
XML | Extensible Markup Language (XML) is a simple, very flexible text format derived from SGML (ISO 8879). Originally designed to meet the challenges of large-scale electronic publishing, XML is also playing an increasingly important role in the exchange of a wide variety of data on the Web and elsewhere. |